Tokens are the fuel of the Decentralized economy and Token Economics, or Tokenomics is at the core. There is a global transformation taking place, and a New Killer App is appearing. The New Killer App? - Web3 and Customer Experience (CX). This is going to be big! - Michael Noel I am … Continue Here about Tokenomics is not Economics – Digital CX -The Digital Transformation Chapter 1
Main Content
Ready to get Started?
- No More Back-and-Forth Emails. Schedule an introductory meeting with one click right here https://calendly.com/bizbuildermike/15min
or message me on https://Linkedin.com/in/MichaelNoel .
Thank you for your interest and I look forward to connecting.
– Michael Noel
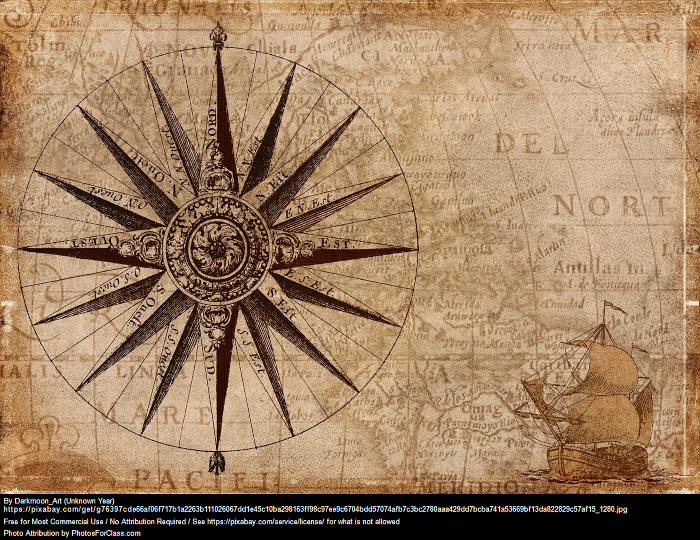
Tokenomics is not Economics – Digital CX -The Digital Transformation Chapter 2
Chapter Two Tokenomics is not Economics the WAMNET In Chapter One we established a few things, such as, Next, we will describe in detail, this smart network, as we introduce the WAMNET, a Wireless, Autonomous, Massive Network, using alternative persistent DNS, and persistent … Continue Here about Tokenomics is not Economics – Digital CX -The Digital Transformation Chapter 2
Tokenomics is not Economics – Digital CX -The Digital Transformation Chapter 3
g Tokenomics is not Economics Chapter Three First Use Case EcoTours.Travel In Chapter Two, we established a few things, such as, Chapter 3 Use Case One - EchoTours.Travel - Customer experience is the challenge of the recovery in Travel and Tourism. Company Name Echo Tours and Travel Website URL … Continue Here about Tokenomics is not Economics – Digital CX -The Digital Transformation Chapter 3
Tokenomics is not Economics – Digital CX -The Digital Transformation Chapter 4
Chapter 4 Moving from Pipes to Platforms Thank you Sangeet Paul Choudary Tokenomics is not Economics Chapter Four from pipes to platforms. In Chapter Three, we established a few things, such as, Problems solved with this Platform First Approach? Chapter 4 Moving from Pipes to Platforms with Sangeet Paul … Continue Here about Tokenomics is not Economics – Digital CX -The Digital Transformation Chapter 4
GBA Speaker Amelia Powers Gardner Utah County Commissioner
Amelia Powers Gardner is currently a Utah County Commissioner. Amelia has over 15 years of experience in the private sector working in engineering, manufacturing, and business development.
She is an elected official, community leader, business consultant, disruptive technology advocate, government innovation leader, and mentor for career women. “If a government agency hasn’t innovated in the last decade, they’re not saving taxpayer dollars, they’re costing you by not innovating.” Amelia Powers Gardner Join us for the Blockchain and Sustainable Economic Growth conference on May 25–27 in Washington, DC at the historic Mayflower hotel. Learn about the latest in blockchain technology from the top minds in blockchain Connect with both local and international government blockchain leaders Connect with businesses and investors interested in blockchain technology Enjoy a fun & exciting evening reception with fellow inventors and pioneers Amelia Powers Gardner